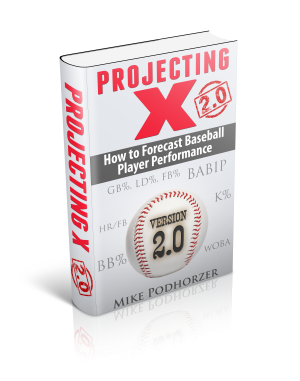
Yes, that’s right. Mike Podhorzer has just released Projecting X 2.0. And I’m excited to announce an updated Projecting X Excel template has been upgraded to be more helpful than ever and has been updated to be consistent with all the new projection methodologies used in Projecting X 2.0.
NOTE: The Projecting X 2.0 Bundle has been updated for the upcoming 2017 MLB season.
What’s New in Projecting X 2.0?
While I would not consider version 2.0 to be a complete re-write of the original Projecting X, it’s certainly an improvement of the process, methods, and formulas used in the original book.
Don’t get me wrong, I love the Projecting X approach. But I did feel there were a couple of methods in the original version that I thought had room for improvement. For example, I’ve come to learn that using K% is superior to using K/9. And I thought the approach to projecting runs and RBI was too subjective.
Well, Podhorzer has addressed all of those issues, improved upon several of his methods, and even introduced new ones.
My favorite changes to the process are:
- A much improved and more scientific methodology for projecting Runs and RBI
- Switching from K/9 and BB/9 to K% and BB%
- A method for projecting quality starts (I get asked about QS projections all the time!!!)
- Addition of metrics like strike percentage (STR%), looking strikes (L/STR), and swinging strikes (S/STR) to pitcher projections, and
- Revisions to the projection of stolen base frequency
What’s New in the Excel Template
The Excel template has been updated to be 100% consistent with all the new methodologies and formulas used in Projecting X 2.0. Take a look.
If you’re a user of the Projecting X 1.0 Excel template, the biggest improvements in the file are:
- Addition of career stats
- Addition of a customizable three-year weighted average
- New team hitting and pitching totals that sum as you project
- More league average information
- New links to Baseball Savant, Brooks Baseball, and RosterResource.com
- It’s now easier to add a new player to the spreadsheet
- The Player ID Map is now easily refresh-able so that when I add new players or change player teams, this information updates in your spreadsheet too
Download the Updated Bundle Today
The updated book and spreadsheet are available for the bundled price of $17.99 (they separately sell for $9.99 each). Click the Add to Cart button below to begin the checkout process.
PDF (recommended) | ![]() |
AZW3 (Kindle) | ![]() |
EPUB (Nook, Apple iPad/iBooks, Sony Reader, Kobo) | ![]() |
![]() |
Continue reading “Now Available – The Projecting X 2.0 Bundle!”