This year’s edition of The Process is now available!
About the Book
A very thorough and detailed write-up of what’s included in the book is available here. At a high level, this book is everything Jeff Zimmerman and I know about how to play rotisserie baseball (and even points leagues). Regardless your level of experience, I guarantee it includes pages and pages of unique ideas, research, and data you have never seen before. We continue to pour our new ideas, new research, and recent realizations into it. The e-book is 265 pages and includes 58 additional pages of appendices full of projections, statistics, and helpful information.
The paperback edition of the book can be purchased from Amazon.com by clicking here.
Want more detail about the topics covered in the book? You can see the table of contents here.
Want a testimonial that the book is this thorough and contains the value we say? You can read a Clay Link’s (RotoWire, 2018 TGFBI Overall Champion) introduction to the 2020 edition here. Or you can see what many other respected fantasy analysts (Rob Silver, Rudy Gamble, Eno Sarris, Mike Podhorzer, Mike Gianella, Tim Heaney) had to say about the 2019 edition here.
My Favorite New Part
Keep reading for details on all that was added to this 2020 edition, but my favorite addition is a detailed study that performed on the 2018 NFBC Main Event, including all the player adds, player drops, and final standings of all 34 leagues and 510 teams. The study sought to confirm if the advice and strategies in the book can be observed and corroborated in the actions of the game’s best managers. The NFBC Main Event, with its $1,700 buy in, prestigious name, and overall competition aspect, offers the best laboratory to study this.
That new study delves into many topics:
- How much does draft position affect the chances of winning a league?
- Do better owners just accrue more playing time? Or are their players also accumulating more stats per AB and per IP? How much more?
- How many free agent transactions do the best teams make?
- How do these better owners spread their transactions throughout the season?
- How do these better owners allocate their FAAB spending?
- What bidding patterns can be observed from winning teams?
- How do these owners allocate transactions between hitters and pitchers?
- How often do these owners acquire two-start pitchers? Closers?
- How much season-long value do these owners acquire and drop during the season?
- How much weekly value do these owners acquire and drop during the season?
For example, here’s a table of data in the section analyzing how owners finish in the standings and the amount of moves spent on closer speculation.
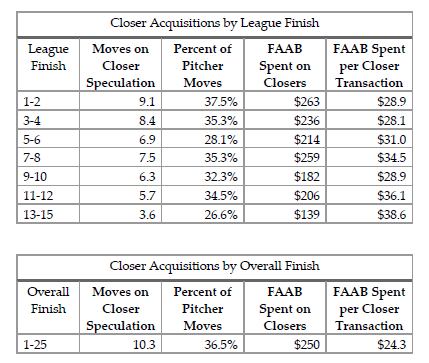
A Personal Note
I’m really proud of this book. Or shall I say, this annual publication that we’ve started. But the intent to have annual editions creates a significant challenge. The book’s main strength is its long-term nature. Much of what we discuss are fundamental concepts to success at rotisserie baseball. Those ideas are not going to change much from year-to-year. It’s certainly going to be a test to keep the book relevant and worthy of your time and money. We understand that.
I am confident we can do this. Afterall, I’ve been writing on this site for several years now, exclusively with a long-term slant to my analysis. I don’t have much time to write. So I choose to focus my efforts doing research, writing instructions, and building tools that will have long-term benefits. I simply don’t have time to devote to writing short-term pieces that will be irrelevant within weeks or even days. Despite blogging for several years and having written a handful of books, I still feel as though we’re finding new ways to play and think about rotisserie baseball. I see no shortage of strategy-related questions to research.
You can see this in the topics we expanded in this year’s book. Among the higher-level strategy questions approached in this year’s book are:
- Are rookies more volatile than established hitters? Does a rookie’s upside offset the possible downside? Do rookie projections differ from the projections of MLB regulars?
- How much does a player’s previous levels of fantasy-production affect their future performance? Do these players offer a higher return on investment than those that have never before attained a given production level?
- What are the key differences owners should know about the SGP and z-score player valuation models? Where are these systems similar?
- How does the cost of closers during the draft compare to their cost in free agency (FAAB)? How does the cost of starting pitchers differ?
- How much value should multi-position eligibility add to a player’s valuation? (Note: This topic was included last year, but we expanded our thoughts)
- Having studied how weekly values appear in 12-team leagues during the 2017 season, how much did things change in 2018? How does the appearance of weekly values change in a 15-team league?
- What do average weekly statistics lines look like for players? For example, what does a $30-35 weekly hitter line look like? What do valuable weekly pitcher statistics look like?
- What strategies and behaviors can we observe from the NFBC’s amazing data (standings, adds, drops, etc.)? What behaviors lead to success? What beliefs about how to best play rotisserie baseball can we confirm by studying this data?
On top of all this, Jeff and I continue to evolve the way we play the game and we share those changes and decisions with you. Here are the new discussions of strategies, tips, and tools included this year:
- We co-owned a team together this season. We share what we learned from this experience, both during the draft and in-season. Our general recommendation is that partnering is very helpful, especially for leagues where there is a grind of weekly transactions.
- To that end, we outline the weekly FAAB process we went through together on that shared league. Having a consistent weekly routine is the key to uncovering valuable players and to avoiding under- or over-bidding. We share the process that works for us.
- We outline the specific tools we use to identify FAAB targets and two-start pitchers. We also share the process we go through for setting lineups and finding important last-minute MLB news.
- We explored different approaches to dealing with catchers this past season. We share the results of those strategies.
- We share a FAAB binning strategy that helps owners stay disciplined about overbidding and maintaining a healthy weekly budget.
Do you have to read through the entire book hunting for what’s new? No! We kept track for you. You can see a full list of changes and jump right to the updated content. Here’s what that list looks like.
Please Click the ‘Buy Now’ Button Below to Purchase the e-Book for $17.99
After clicking the “Buy Now” button, you’ll be taken through an online checkout process using PayPal. There is also an option to pay with a debit or credit card. After completing the purchase, a link to download the PDF book will immediately be e-mailed to you. You can read the PDF on any mobile device, PC, or tablet.
Or Click Below to Buy the Paperback Edition at Amazon for $22.99
Click here to buy The Process – 2020 Edition: Integrating Valuations and Biases into a Winning Fantasy Baseball Formula at Amazon.com.